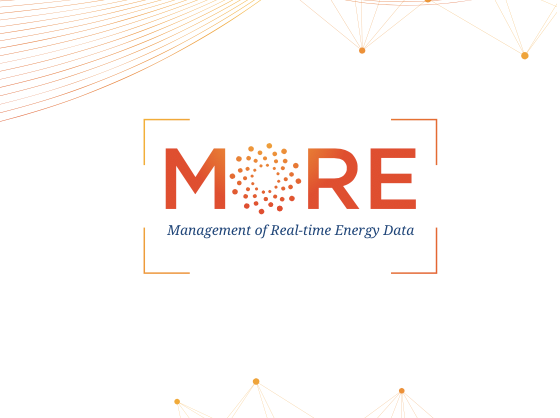
MORE | Management of Real-time Energy Data - IT, big data and machine learning challenges in time series data - Renewable Energy Sources (RES) sector | Management of Real-time Energy Data
Format: Online, Zoom
Date and Time: March 14, 2022, 1 PM - 3:30 PM GMT
Duration: 2.5 hours
MORE | Management of Real-time Energy Data - About the event
You are cordially invited to the bi-annual IBM-Athena colloquium series on the industry challenges due to the exponential growth of time-series data. The colloquium series strives to cover the challenges arising from high frequency and/or high volume time series data in various sectors like RES, water, inventory management etc.
The first colloquium aims to understand the challenges in handling big data and machine learning algorithms in renewable energy sources sector. Traditionally, data in RES has been aggregated over 5-10 minute intervals and business use cases were built on this aggregated data for the RES sector. However, with lowering cost of sensors and communications, and increasing demand for high frequency updates and use cases, there is an exponential growth in the data generated by the devices. There are natural challenges of persistence and analytics on this data. Privacy and security add an additional layer of complexity. The colloquium will cover the use cases that arise from high frequency data and the technical challenges to handle this data and provide analytics on top.
The online event takes place between 1 PM - 3:30 PM GMT on March 14, 2022. Speakers and panelists are international industry and academic experts that would provide a holistic viewpoint on the future of computing in the RES sector.
Participation is free and open to all, but you need to register here:
RegisterMORE | Management of Real-time Energy Data - Agenda for the colloquium is as below:
Speaker: Dhaval Salwala
Title: MC to open
Time: 1:00 PM-1:05 PM
Duration: 5 minutes
Speaker: Prof. Ioannis Emiris
Title: Executive speaker with open remarks
Time: 1:05 PM-1:10 PM
Duration: 5 minutes
Speaker: Dr. Shivkumar Kalyanaraman CTO, Energy & Mobility, Microsoft R&D India (& Azure Global)
Title: The Software- and AI-Driven Future of Renewables
Time: 1:15 PM-1:45 PM
Duration: 30 minutes
Title: IBM Pre-doc program mini-talks
Time: 1:45 PM-1:55 PM
Duration: 10 minutes
Speaker: Dr. Paul Poncet (ENGIE, Head of Data Science for the Darwin Platform)
Title: Optimizing operation and maintenance of renewable energy assets: some machine learning challenges
Time: 1:55 PM-2:30 PM
Duration: 35 minutes
Speaker: Prof. Themis Palpanas
Title: Easy, Accurate, and Fast Complex Analytics on Big Data Series
Collections: Renewable Energy Sources and Beyond
Time: 2:30 PM-3:00 PM
Duration: 30 minutes
Speaker: Dr. George E. Konstantoulakis (Inaccess), Prof. Themis Palpanas, Dr. Seshu Tirupathi (IBM Research Europe)
Title: Panel discussion
Time: 3:00 PM-3:30 PM
Duration: 30 minutes
Speaker | Title | Time | Duration |
---|---|---|---|
Dhaval Salwala |
MC to open |
1:00 PM-1:05 PM | 5 minutes |
Prof. Ioannis Emiris |
Executive speaker with open remarks |
1:05 PM-1:10 PM | 5 minutes |
Dr. Shivkumar Kalyanaraman CTO, Energy & Mobility, Microsoft R&D India (& Azure Global) |
The Software- and AI-Driven Future of Renewables |
1:15 PM-1:45 PM | 30 minutes |
IBM Pre-doc program mini-talks |
- | 1:45 PM-1:55 PM | 10 minutes |
Dr. Paul Poncet (ENGIE, Head of Data Science for the Darwin Platform) |
Optimizing operation and maintenance of renewable energy assets: some machine learning challenges |
1:55 PM-2:30 PM | 35 minutes |
Prof. Themis Palpanas |
Easy, Accurate, and Fast Complex Analytics on Big Data Series |
2:30 PM-3:00 PM | 30 minutes |
Dr. George E. Konstantoulakis (Inaccess), Prof. Themis Palpanas, Dr. Seshu Tirupathi (IBM Research Europe) |
Panel discussion |
3:00 PM-3:30 PM | 30 minutes |
MORE | Management of Real-time Energy Data - Speakers Details:
Shivkumar Kalyanaraman
The Software- and AI-Driven Future of Renewables
Deep decarbonization and the rapid electrification of energy will require greater penetration of renewables. As renewables penetration crosses 10-20% of the grid electricity demand (and other supply sources correspondingly adjust), the intermittency and volatility of renewable supply will increasingly dominate. Renewable supply and grid electricity demand matched via a combination of multiple markets, energy storage and an orchestrated portfolio of flexibility resources. The future of renewables will fundamentally be driven by software and AI on the cloud to manage this transition. This talk will unwrap the various challenges and opportunities around this transition.
Profile
Dr. Shivkumar Kalyanaraman is CTO, Energy & Mobility, Microsoft R&D India for Azure Global. Previously Shiv was Executive General Manager of Growth Offerings at GE PowerConversion responsible for new Line of Business development in e-Mobility, Commercial &Industrial Solar and digital/AI innovations. Earlier he was at IBM Research - India, and theChief Scientist of IBM Research - Australia. Before IBM, he was a tenured Full Professor atRensselaer Polytechnic Institute in Troy, NY, USA. Shiv has degrees from Indian Institute ofTechnology, Madras (B.Tech), Ohio State University (MS, PhD) and RPI (Executive MBA).Shiv is a Distinguished Alumnus Awardee of IIT Madras & Ohio State University(2021), Fellow of the IEEE (2010), Fellow of Indian National Academy of Engineering(2015), ACM Distinguished Scientist (2010), MIT Technology Review TR100 younginnovator (1999).
Themis Palpanas
Easy, Accurate, and Fast Complex Analytics on Big Data Series
Collections: Renewable Energy Sources and Beyond
There is an increasingly pressing need, by several applications in diverse domains, for developing techniques able to manage and analyze very large collections of sequences, or data series. Examples of such applications come from various monitoring applications, including in power utility companies, where we need to apply machine learning techniques for knowledge extraction. It is not unusual for these applications to involve numbers of data series in the order of hundreds of millions to billions, which are often times not analyzed in their full detail due to their sheer size. However, no existing data management solution can offer native support for sequences and the corresponding operators necessary for complex analytics. In this talk, we describe our efforts in designing techniques for indexing and analyzing truly massive collections of data series that enable scientists to run complex analytics on their data. These techniques are orders of magnitude faster than the state of the art, and are applied, among others, on datasets derived from operation monitoring applications (e.g., from sensors on wind turbine farms), as well as remote sensing applications with the purpose of guiding predictive maintenance and operational optimization pipelines.
Profile
Themis Palpanas is Senior Member of the French University Institute (IUF), a distinction that recognizes excellence across all academic disciplines, and professor of computer science at the University of Paris (France), where he is director of the Data Intelligence Institute of Paris (diiP), and director of the data management group, diNo. He received the BS degree from the National Technical University of Athens, Greece, and the MSc and PhD degrees from the University of Toronto, Canada. He has previously held positions at the University of California at Riverside, University of Trento, and at IBM T.J. Watson Research Center, and visited Microsoft Research, and the IBM Almaden Research Center. His interests include problems related to data science (big data analytics and machine learning applications). He is the author of 9 US patents (3 of which have been implemented in world-leading commercial data management products), and 2 French patents. He is the recipient of 3 Best Paper awards, and the IBM Shared University Research (SUR) Award. He is currently serving on the VLDB Endowment Board of Trustees, as an Associate Editor in the TKDE, and IDA journals, as well as on the Editorial Advisory Board of the IS journal, and the Editorial Board of the TLDKS Journal. He has served as Editor in Chief for the BDR Journal (that he drove to an impact factor of 3.578 and cite score of 8.6), as General Chair for VLDB 2013, Associate Editor for VLDB 2022, 2019 and 2017, Research PC Vice Chair for ICDE 2020, and Workshop Chair for EDBT 2016, ADBIS 2013, and ADBIS 2014, General Chair for the PDA@IOT International Workshop (in conjunction with VLDB 2014), and General Chair for the Event Processing Symposium 2009.
Paul Poncet
Optimizing operation and maintenance of renewable energy assets: some machine learning challenges
Relying on the experience we have with Darwin, Engie’s digital platform that provides software solutions on top of time series collected from renewable assets in operation, we shall first give an overview of data science and predictive maintenance use cases we have been facing so far. Then, we shall reflect on the kind of machine learning.
Profile
Paul Poncet has 15 years of experience in the Energy industry; he is currently leading a team of data scientists and data engineers at Engie Digital who invent, develop, and deploy algorithms for the renewable energy businesses. He owns a Master degree in Engineering from Ecole des Mines de Paris, and a PhD in Applied Mathematics from Ecole Polytechnique.